Can complex datasets reveal hidden truths, guiding decisions and shaping our understanding of the world? Exploratory Data Analysis (EDA) provides the lens through which these complex datasets are examined, transforming raw numbers into actionable insights.
At its core, EDA is a methodical approach to analyzing data, going beyond simple number crunching to uncover patterns, trends, and relationships. Using a combination of statistical tools and visual representations, EDA allows analysts to explore data thoroughly, identify anomalies, and formulate hypotheses that can then be tested rigorously. It's the initial exploration phase, the detective work that precedes more formal statistical modeling.
The process itself involves a variety of techniques, from calculating descriptive statistics like mean, median, and standard deviation to creating visualisations such as histograms, scatter plots, and box plots. Each method offers a different perspective on the data, allowing analysts to build a comprehensive understanding of its structure and characteristics. This is crucial to detect errors, identify outliers, and understand the distribution of values within the dataset.
- Mastering Ssh Over The Internet With Raspberry Pi The Ultimate Guide
- Iot Vpc Networks The Future Of Secure And Scalable Connectivity
EDA's roots can be traced back to the early days of computing, where the need to understand large datasets became increasingly apparent. The evolution of EDA tools and techniques has mirrored the growth of computing power and the increasing availability of data. Today, EDA is an essential component of data science, machine learning, and any field that relies on data-driven decision making. From financial analysis to scientific research, from marketing strategy to healthcare, the ability to explore and understand data through EDA is a valuable skill.
EDA goes beyond simply presenting data; it encourages the user to interact with it. This interactive approach allows for a deeper level of understanding, which in turn, informs more effective decision-making. EDA is not just about finding the answers, but also about asking the right questions. By iteratively exploring the data, analysts can refine their understanding and uncover insights that may not have been immediately apparent.
While EDA doesn't directly address the hardware level complexities of chip manufacturing, it plays a vital role in ensuring semiconductor products meet desired performance and density levels. EDA tools are used to aid the design and verification stages of the manufacturing process. Much like the importance of EDA in other fields, ensuring a robust methodology is key to understanding the data that is being used to create designs. Also, the design process will allow for more optimized chip designs and less risk.
- Erome Jameliz A Rising Star In The Music Industry
- Free Download Remote Iot Platform Ssh For Raspberry Pi
The importance of EDA continues to evolve as the volume, variety, and velocity of data increase. As data becomes more complex, the need for sophisticated EDA techniques and tools grows. The constant evolution of the data landscape demands that EDA practices are updated. This ensures that they remain effective in exploring and understanding the ever-expanding universe of information. In turn, this helps to develop strategies that lead to actionable insight.
The EDA process is often classified into two main methods:
- Univariate Analysis: This focuses on examining a single variable at a time. The goal is to understand the distribution of values for each variable, identifying measures of central tendency and spread. Visualizations such as histograms and box plots are commonly used in univariate analysis.
- Multivariate Analysis: This involves exploring the relationships between two or more variables. Techniques include scatter plots, correlation analysis, and more complex methods such as regression analysis and cluster analysis.
The world of EDA is not static; it's constantly evolving. New tools and methods are always emerging. Data scientists and analysts are embracing these new developments to stay ahead of the curve. This constant advancement allows for even more in-depth exploration of data and allows us to refine techniques for analyzing data.
In the realm of gardening and landscaping, EDA finds another application, though in a less technical and direct manner. It helps to ensure that the right kind of product is available for any customer. For example, the need for a robust and elegant container to brighten up a balcony or terrace will be addressed by examining the available options. This involves a wide range of flowerpots that have been created for gardens, balconies, terraces, and interiors.
Similarly, the "eda portal" serves as a web platform, which supports the processing of energy-related processes for energy service providers and energy communities. The portal offers many sizes and colors to make certain that the ideal pot can be found to showcase plants.
Aspect | Details |
---|---|
Definition | Exploratory Data Analysis (EDA) is a key step in data analysis, focusing on understanding patterns, trends, and relationships through statistical tools and visualizations. |
Purpose | Summarize main characteristics of a dataset, identify patterns, anomalies, and relationships within the data. |
Methods |
|
Tools | Statistical graphics (histograms, scatter plots, box plots), descriptive statistics (mean, median, standard deviation), and data visualization techniques. |
Applications | Data science, machine learning, business analytics, scientific research, financial analysis, and any field relying on data-driven decision-making. |
Relevance | Crucial for understanding data, identifying potential issues, and formulating hypotheses for further analysis. It is the foundation of effective data-driven decision-making. |
Historical Context | Emerged with the increase of computing power and data availability. Developments are aligned with the growth of computational capabilities. |
Example | Using a scatter plot to analyze the relationship between marketing spend and sales revenue. |
Benefits |
|
Limitations | EDA is exploratory, not confirmatory. Results are suggestive, not definitive. It may not be suitable for very large datasets without proper tools. |
EDA in Semiconductor Manufacturing | EDA tools are used in the design and verification process of semiconductor manufacturing. This helps ensure the semiconductor products meet the required performance. |
Evolution | EDA has undergone constant evolution along with the data landscape, new tools, and methods are constantly emerging to keep up with more complex datasets. |
Related Concepts | Data visualization, statistical analysis, data mining, data wrangling. |
Future | EDA will continue to be essential. The rise of big data, machine learning, and AI makes it a crucial part of how organizations use data to make informed decisions. |
For further information, you may refer to the following website:
Example EDA Resource
In conclusion, EDA is a foundational process. It underpins data-driven decision-making across various domains. As the volume and complexity of data increase, the importance of EDA will only grow. Its ability to uncover patterns, insights, and relationships makes it an indispensable tool. As the field continues to evolve, EDA professionals will need to adapt and learn new techniques. This will help them make better informed decisions.
- Hdhub4u Movie Your Ultimate Destination For Highquality Movies
- Iot Core Remoteiot Display Chart Your Ultimate Guide To Realtime Data Visualization
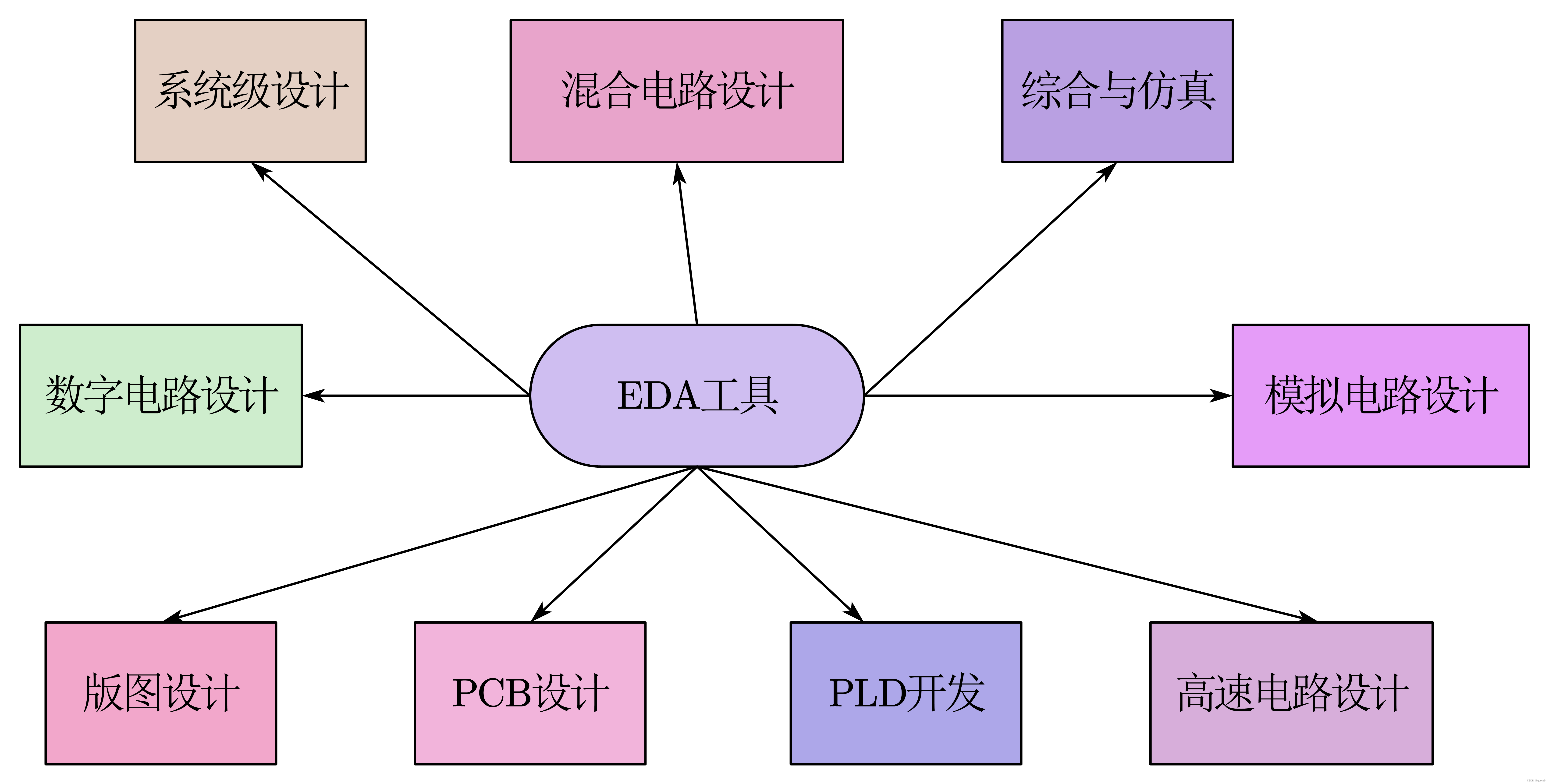

